BGU (Israël) : détection des charançons rouges destructeurs de palmiers grâce à Google Street View
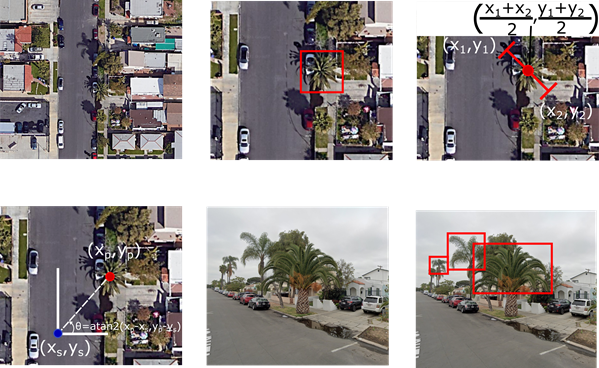
[:fr]
Le charançon rouge du palmier pourrait sans doute être qualifié de pandémie mondiale de palmier. Au cours de la dernière décennie, ils ont migré à travers le monde, pondant leurs œufs dans les arbres afin que leurs larves puissent grignoter l’arbre pendant leur croissance. Les tunnels qui en résultent endommagent et affaiblissent les arbres, provoquant même la rupture et l’effondrement de certains arbres. Les dommages causés aux cultivateurs de dattes, ainsi qu’aux palmiers utilisés comme éléments décoratifs dans les quartiers du monde entier, sont monumentaux. De plus, les charançons peuvent se propager 50 kilomètres par jour, une détection précoce est donc essentielle.
Rien qu’en Espagne et en Italie, la lutte antiparasitaire et le remplacement des arbres va atteindre 200 millions d’euros en 2023 selon l’Organisation des Nations Unies pour l’alimentation et l’agriculture (FAO). On trouve des charançons dans 85 pays et régions du monde entier, selon l’Organisation européenne et méditerranéenne pour la protection des végétaux (OEPP). À ce jour, la seule méthode pour déterminer si les charançons ont infesté un arbre est l’évaluation visuelle et acoustique de chaque arbre. Un tel processus est laborieux, prend du temps et n’est pas pratique pour une surveillance municipale à grande échelle. Le Dr Michael Fire travaille au Département de génie logiciel et des systèmes d’information à l’université Ben Gurion du Néguev et il dirige le laboratoire Data4Good lab.
Le Dr Michael Fire réside dans une petite ville qui compte plusieurs palmiers dans presque toutes les rues. Il en a même un dans sa cour, et a découvert que la municipalité n’avait aucune trace de cet arbre. La municipalité a envoyé quelqu’un pour détruire l’arbre pour l’empêcher de s’infecter. Pour la plupart des gens, ce serait la fin de l’histoire de son « cher arbre ». Mais le Dr Fire est sorti des sentiers battus.

« J’ai commencé à penser, et si je pouvais aider la municipalité en développant un moyen pour eux de surveiller tous les palmiers ? » explique le Dr Fire. Le Dr Fire a expliqué son problème d’arbre isolé à son équipe, Dima Kagan et le Dr Galit Furhmann Alpert, et ils ont conçu une solution de surveillance globale. Tout d’abord, ils ont collecté des images Google aériennes et de rue de palmiers. Ensuite, ils ont formé trois modèles d’apprentissage deep learning : un pour détecter les palmiers dans des images aériennes, un pour les détecter dans des images de vue de la rue et un troisième pour répertorier les palmiers infectés.
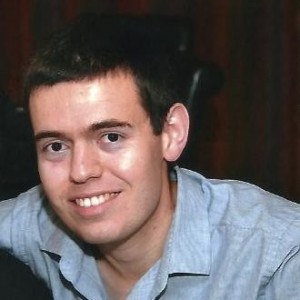
Ils ont analysé plus de 100000 images pour construire leurs modèles. Ils ont testé leur système de détection sur des photos de San Diego, en Californie, car ils savaient qu’il y avait eu une infestation là-bas en 2016. Ils ont pu trouver trois des quatre arbres infectés notés dans une étude de 2016. Ils ont également réussi à localiser des arbres dans Israël et dans le comté de Miami-Dade en Floride. Leurs résultats ont été téléchargés sur Arxiv, l’archive numérique en ligne pré-imprimée. Leur système peut détecter les infections sévères et moyennes. Un perfectionnement à venir pourrait conduire à une détection plus précoce, écrivent les chercheurs.
Publication on Arxiv, avril 2021
Traduction/adaptation Esther Amar pour Israël Science Info
[:en]

To date, the sole method of determining whether weevils have infested a tree has been a visual and acoustic assessment of individual trees. Such a process is laborious – time consuming and impractical for large-scale municipal monitoring. Dr. Michael Fire works at the Department of Software and Information Systems Engineering (BGU) and head of the Data4Good lab. Dr. Fire lives in a little town with multiple palm trees on almost every street. In fact, Dr. Fire has one in his front yard. He knew he had one, but the municipality had no record of such a tree, Dr. Fire discovered. The municipality did send someone to spray the tree to prevent it from infection. For most people, that would be the end of the story – my tree protected. But Dr. Fire is not most people. « I started thinking, what if I could help the municipality by developing a way for them to monitor all of the palm trees? » explains Dr. Fire.
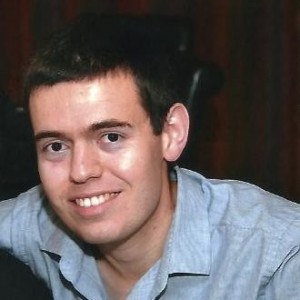
Dr. Fire took his individual tree problem back to his team, Dima Kagan and Dr. Galit Furhmann Alpert, and they devised a global monitoring solution. First, they collected Google aerial and street view images of palm trees. Then, they trained three deep learning models: one to detect palm trees in aerial images, one to detect them in street view images and a third – an infected palm classifier. They analyzed more than 100,000 images to build their models. They tested their detection system on photos of San Diego, CA because they knew there had been an infestation there in 2016. They were able to find three out of four of the infected trees noted in a study from 2016. They also successfully located trees in Israel and in Miami-Dade county in Florida.
[:]